If there is one thing that the pandemic is bound to teach humanity, it is the will to go with the flow and not plan too ahead in life. That said, counterintuitively, it seems important to be prepared and planned when similar outbreaks arise in the future.
A healthcare startup from Canada, BlueDot, in its report, shared that the outbreak was fast spreading in China even before the World Health Organisation (WHO) had reported it. The startup’s outbreak risk software helps mitigate the exposure to infectious diseases using Artificial Intelligence.
It goes to show that healthcare organisations, governments, and public health officials would have to rely on these AI-based systems in the future to prevent similar outbreaks from reoccurring.
The usage of digital healthcare devices spanning across smartphones, wearables, internet applications, and social networks is a broad spectrum in itself. The idea around monitoring an individual’s health status and well-being, powered by data and patient information, has now become a norm.
AI in the frontline of redefining the health ecosystem
With so much attention around using AI in healthcare, there is only a small set of stakeholders that truly understand the real impact and application of it.
The clear macro-level problems in healthcare, some of which are decades-old and traditional, include:
- Lack of access to affordable and quality healthcare
- Inadequate resources, skewed supply and demand system, and distribution
- Lack of standardisation, error-prone diagnosis, unevolved care management
- Rising costs, poor healthcare coverage, low penetration by insurance
AI has been playing a major role in supercharging the fields of drug discovery, by simulating a wide variety of models and experiments on computers. Simply putting it, AI could dress off as an engine sitting on top of enormous amounts of patient data, generating relevant insights to all stakeholders and churning out effective clinical decisions.
With this, the involved peers would be able to be better at underwriting risks and customise the relevant offerings. This also allows pharma research labs to be much efficient at drug discovery and development.
- Some examples include bridging the gaps in acute radiology and the subsequent report annotation and generation through tele-solutions deep learning.
- Using images in pathology, AI helps generate precision-driven diagnosis.
- With something as simple as our everyday push notifications and digital nudges, AI has its hands all over treatment for chronic conditions like obesity, hypertension and diabetes.
The focus areas for Accel are AI in diagnostics, drug discovery, precision medicine and digital therapeutics with some recent investments made in these categories. The importance of the applications of AI and the large industry spend are the key attributes to double click on these categories.
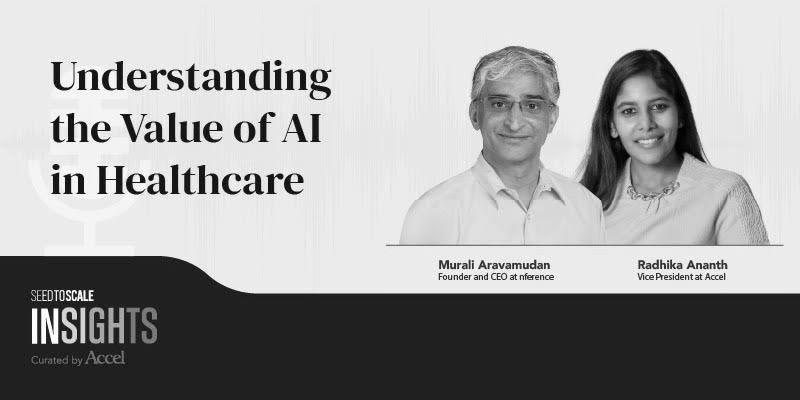
Murali Aravamudan, Founder and CEO at nference, in conversation with Radhika Ananth, Vice President at Accel
Making bio-medical knowledge computable
In biology, scientists have traditionally shared insights and knowledge through written papers in the long-form format. The whole objective around making sense of all this knowledge and literature is directly contributed by deep learning techniques.
When deep learning is applied to text in automatic translation at companies like Google and Facebook, auto-encoding and self-supervised neural networks provide a wide range of meaningful insights.
Murali Aravamudan, CEO and Founder of nference said, “The digitisation of biology and electronic medical care records, coupled with the explosion of scientific literature led me to identify that I could solve meaningful problems of human life. This is the century of biology, and we need to conquer the subject of it fully with all the scientific advancements coming out there.”
Nference.ai is a Cambridge-based company that partners with various biopharma companies to solve the numerous problems in drug discovery, clinical research, life cycle management, clinical operations and commercial strategy. The comprehensive software platform converts a vast array of biomedical knowledge into computable data, resulting in answers to complex questions around disease biology and treatment outcomes.
Murali says, “With the help of Artificial Intelligence (AI) in human genome sequencing and digital healthcare, along with clinical healthcare records, a massive digital explosion is poised to occur in the global healthcare ecosystem. This resembles the Cambrian radiation explosion that happened 540 million years ago, but all for the greater good.”
The applications of AI in the future
Before the COVID-19 pandemic, it would have been unimaginable to detect the symptoms of a certain virus infection like this. When SARS first broke out, it took a few years to make sense of all the symptoms when a virus like this infects the human body.
But during COVID-19, it took only a matter of a few weeks for healthcare organisations like nference and Mayo Clinic to take data from past virus outbreaks like Influenza, 2003 SARS, and detect common symptoms like lack of sense of smell and taste.
A range of new sensory digital devices would now add to the existing human phenotypes and that makes it possible to predict any possible future health conditions. Intervention and diagnosis becomes easier as part of preventive healthcare, thanks to the applications of AI in digital health.
- When it comes to genome sequencing, the primary idea is to identify whether the target molecules in the DNA structure would modulate or not.
- Clearly, the problem is within the exponential searching within the gene structures to narrow down millions of preclinical molecules into mere five molecules that’s worth advancing.
- In the next stage, when the pre-clinical molecules move on to animal-model testing, lead optimisation comes into picture which also has its own set of unknown variables.
- From animal-model testing to phases one, two, three of pharma testing, artificial intelligence (AI) plays a major role across every stage.
Murali explains, “The way I see it, there are no limitations as to how AI finds its applications in the lifecycle management in the pharmaceutical industry. As care becomes virtual with telemedicine, whether it is acute care or chronic care, the lifecycle shrinks down to a matter of a few years, which would be decades without using AI.”
Challenges while adopting AI in Healthcare
There are naturally a whole plethora of challenges while trying to adapt to new technology within a system. However, healthcare entrepreneurs are highly optimistic of a certain massive disruption in the near future. Though it takes much longer than anticipated, what is underestimated is the scale it reaches when the trend catches up at the appropriate time.
- The idea of transparency and how explainable AI can get to the doctors ecosystem is going to be a challenge, to be able to convince them to move on from legacy systems. Startups partnering with pharmas and working collaboratively with physicians will support in building credibility, trust and validation of the technology.
- The technological possibilities of AI should not be oversold without peeling down all the underlying layers of it. Various diverse sets of systems would have to be built in order to avoid the possible bias by the underlying probability distribution, but rely more on reasoning.
- These above mentioned factors would have to be working in tandem with the ecosystem physicians and medical practitioners. The idea is to get the ecosystem comfortable with the idea of machines helping augment the decision-making mechanism in healthcare, with as much of seamless integration into existing workflows as possible.
Anand Daniel is a seed/early-stage venture investor with Accel Partners.
Edited by Kanishk Singh
(Disclaimer: The views and opinions expressed in this article are those of the author and do not necessarily reflect the views of YourStory.)
Link : https://yourstory.com/2021/01/accel-podcast-artificial-intelligence-healthcare
Author :- Anand Daniel ( )
January 03, 2021 at 06:15AM
YourStory